AI Patient Summary
Background
Clinicians face an overwhelming volume of complex, machine-oriented healthcare data, making it both time-consuming and challenging to navigate and interpret efficiently.
Overview
Particle solves for this challenge by leveraging large language models (LLMs) to convert medical records—both structured and unstructured, including clinical notes—into concise, focused summaries. These summaries deliver a quick yet comprehensive overview of the most critical information, ensuring a complete clinical picture at a glance.
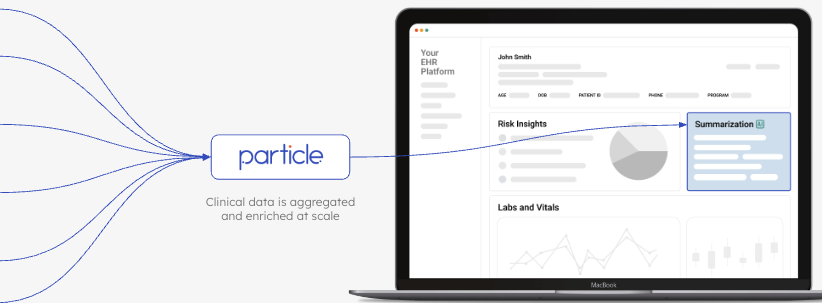
Key insights are synthesized and surfaced from comprehensive, clinical records.
Value Add
Snapshot's AI Summary is a simple and efficient tool designed to synthesize comprehensive patient records into a clear, readable format. This summary supports a variety of use cases, including:
- Analyzing Recent Lab Trends: Quickly identify changes and patterns in lab results.
- Streamlining New Patient Onboarding: Reduce physician review time and enhance onboarding efficiency.
- Chronic Care Program Enrollment: Identify eligible patients for targeted chronic care initiatives.
- Closing Gaps in Care: Pinpoint and address care gaps to improve patient outcomes.
With Snapshot's AI Summary, transform complex patient data into actionable insights with ease.
Hart Fallon
Using our synthetic sandbox patient - Hart Fallon - see an example of the AI Summary below!
Clinician Focused Summaries
Patient Persona First
AI starts by building a comprehensive patient profile with focused “contextual search” for chronic conditions, major surgeries, and key health events.
Adopt Clinical Specialization
The AI then dynamically assumes the perspective of most relevant clinical specialty, focusing on data specific to that field, beyond what a general clinician might think to search for.
Critical Data Extraction
Guided by the clinical specialty, the AI extracts key data and flags missing information specific to that specialty, including rare conditions, niche procedures, or specialized lab markers.
Thought-chaining and Reasoning
The AI uses an "agentic" workflow to iteratively improve summaries and self-heal errors. Reasoning triggers force it to reread the prompt, encouraging step-by-step thinking instead of regurgitation, refining outputs to reach an ideal state.
Customizable Clinical Summaries
Our AI offers both Ontological and Problem-Oriented Narrative formats, easily adapting to any user-specified formatting. Data is organized according to clinical priorities, ensuring it's relevant and actionable for patient care.
How we do it
Data Engineering
Meticulously clean & structure complex patient data into an ontological model that significantly enhances the AI's ability to search, reason, and deliver clinical insights.
AI Engineering
Leverage the latest LLM models, thought-chaining techniques, and “reasoning” triggers to intelligently surface the most relevant information.
Grounding in Maximum Truth
Utilize advanced encoding/retrieval, and the largest context window on the market to 10x capacity, grounding generation in the maximum amount of patient history data possible.
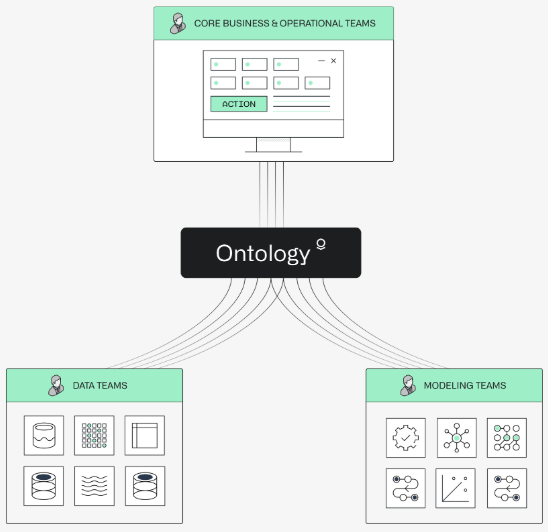
Updated 4 months ago